Farm Progress America, April 26, 2024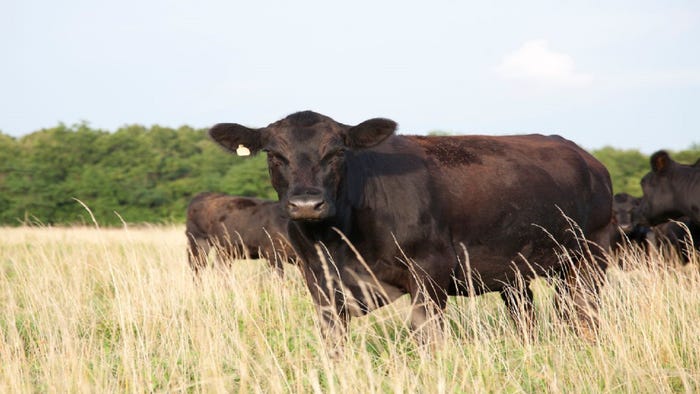
Farming Business Management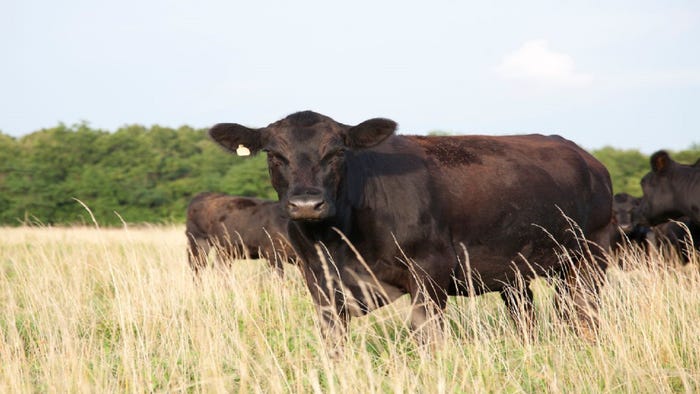
Farm Progress America, April 26, 2024Farm Progress America, April 26, 2024
Mike Pearson takes a look at two new rules coming out of the Department of Interior regarding land use.
Subscribe to Our Newsletters
National Hog Farmer is the source for hog production, management and market news